A Critical Exploration of AI and Human Cognition
Originally submitted as part of a “Cognitive Science” module for an MSc in Human-Computer Interaction at University College Dublin, this essay earned an A+ grade. Professor feedback is provided at the conclusion.
Introduction: Defining Human Thought
Jiddu Krishnamurti once said, “We do not see things as they are, but as we are” (Krishnamurti, 1969, p. 32). This captures the notion that human cognition is inherently self-referential and deeply tied to personal experience, emotion, and social context. Humans interpret the world not as detached observers but through subjective lenses that filter perception and decision-making. In the domains of cognitive science and philosophy, defining “thinking” has long presented a challenge. Thinking involves processes such as reasoning, problem-solving, self-reflection, and consciousness, all of which are heavily shaped by real-world interactions.
Three Foundational Elements of Human Thought
- Embodiment
Human cognition is closely linked to physical experiences. Concepts like “hot” or “weight” do not emerge from static definitions; they stem from tangible sensory and emotional interactions with the environment. - Emotional Influence
Emotion profoundly impacts thinking, shaping decisions, problem-solving, and reasoning. It is impossible to separate cognition from the emotional undertones that guide attention and priorities. - Evolutionary Adaptation
Human cognition developed to address survival challenges, from pattern recognition in nature to navigating complex social structures.
From this perspective, “thinking” is not merely an exercise in abstract logic; instead, it is an embodied, context-based process that evolves through direct engagement with the physical and social world.
Hofstadter’s Framework: Self-Reference and Creative Loops
Douglas Hofstadter’s seminal work, Gödel, Escher, Bach: An Eternal Golden Braid (1979), provides an influential lens for understanding the recursive nature of human cognition. Drawing on Gödel’s incompleteness theorems, Hofstadter illustrates how systems can contain statements that are logically valid yet unprovable within their own frameworks. This paradox reveals the creative potential of self-reference, a quality that appears in human thought.
Self-Reflective Recursion
When a person experiences sadness, for instance, questions arise: “Why am I sad? What caused this feeling? How might this affect future decisions?” Such recursive loops build upon prior layers of thought, creating deeper insights into personal or abstract problems.
Symbol Manipulation and Creative Insights
Human minds also rely on symbols that can represent objects, feelings, or concepts. These symbols follow mental “rules” but can be combined in novel ways, prompting unexpected leaps in understanding or creative breakthroughs. Unlike static rule-based systems, human cognition draws on emotional depth, recursive self-reference, and symbolic reorganization, making genuine innovation possible.
Do Large Language Models “Understand”?
Blaise Agüera y Arcas’s (2022) article, Do Large Language Models Understand Us?, challenges traditional assumptions about machine intelligence by proposing that robust statistical processing can constitute a form of understanding. When Large Language Models (LLMs) such as GPT generate coherent sentences and respond contextually, some observers infer a near-human intelligence. Yet, the question remains: does pattern recognition alone equate to thinking?
Statistical Processing vs. Embodied Cognition
LLMs analyze enormous text datasets, employing probabilistic methods to predict subsequent words. These models can replicate emotionally charged language and form context-relevant dialogue, but they lack the sensory and emotional grounding intrinsic to human thought. For instance, references to “cold” in an LLM’s output rely on textual patterns linking “cold” to “winter” or “snow,” rather than on the physical sensation of shivering or touching ice.
The Problem of Physical Interaction
Human cognitive development involves active learning through multisensory experiences, exemplified by a child who learns “soft” by touching objects. LLMs, conversely, exist exclusively in the realm of text and cannot directly interact with the physical world. As a result, genuine human-like cognition—complete with sensory and emotional dimensions—remains inaccessible to purely text-based models.
Emotional and Social Nuances: The Role of Theory of Mind
Theory of mind, or the capacity to infer others’ thoughts and emotions, is another dimension of human cognition. Agüera y Arcas (2022) suggests that advanced LLMs exhibit a rudimentary version of theory of mind by maintaining conversational consistency or simulating emotion. However, these capabilities are widely viewed as imitation rather than genuine empathy.
Sarcasm, Subtext, and Emotional Resonance
Humans perceive subtext, tone, and nonverbal cues that inform the interpretation of language. LLMs can replicate stylistic elements but typically lack the deeper emotional involvement that stems from living, embodied experiences. Language, for humans, is more than patterns; it is interwoven with feelings, context, and awareness of others’ mental states.
The Centrality of Self-Recursive Thought
Self-recursion enables humans to examine their own thinking processes. By reflecting on personal experiences and emotions, individuals can form metaphors, generate creative works, and produce wholly new interpretations of the world. Hofstadter (1979) describes this as a “strange loop,” in which the mind observes itself, leading to emergent properties such as introspection and creativity.
The Machine Perspective
LLMs are designed to find optimal solutions through extensive data analysis. While they excel at pattern recognition and generating plausible text, they lack the “strange loop” inherent in human cognition. Self-awareness, or the capacity to examine personal thought processes, remains absent from statistical modeling, regardless of how sophisticated the model becomes.
Human Complexity and the Limits of AI
Humans are shaped by emotions, physical interactions, cultural norms, beliefs, and subjective experiences. These multifaceted dimensions lead to spontaneous ideas, empathy, or epiphanies that machines cannot replicate.
Adaptive and Contextual Learning
Babies learn the world’s physics and social rules through trial-and-error, aided by an innate curiosity. While AI researchers study infant cognition to inform machine learning models, LLMs remain text-bound and generally cannot acquire the same intuitive understanding of physical or social realities.
Creativity, Emotion, and Contradiction
Humans often hold contradictory beliefs and exhibit complex emotional responses. Such paradoxes fuel creativity, prompting leaps in innovation and unexpected perspectives. In contrast, AI systems rely on the data provided by humans and remain confined by algorithmic logic, irrespective of how extensive that logic becomes.
Turing’s Legacy and the Nature of Imitation
Alan Turing’s seminal work explored whether machines could imitate human cognition closely enough to pass as truly intelligent (Turing, 1950). Modern LLMs follow this imitation-based framework: they produce human-like responses through statistical patterning rather than possessing subjective awareness. Agüera y Arcas (2022) likens LLMs to philosophical zombies (p-zombies), which demonstrate outwardly human behaviors yet lack an inner experience.
An LLM might draft an apology that appears empathetic, but the model’s function is grounded in probabilistic outputs, not genuine emotional engagement. If humans stopped supplying cultural narratives and emotional data, these AI systems would have no basis for generating meaningful content. This dynamic underlines AI’s nature as a mirror of collective human patterns rather than an originator of independent thought.
Insights from Developmental Psychology
Studying how infants learn sheds further light on why human cognition remains distinct. Infants rapidly grasp abstract concepts such as cause and effect, guided by both sensory interactions and social cues. They accumulate multimodal data—physical, emotional, and social—and adapt in ways that text-based AI models cannot match.
Uniquely Human Adaptability
Humans demonstrate unparalleled adaptability, transitioning from one career or creative pursuit to another based on internal drives or emotional revelations. This freedom to explore, question, and transform is deeply rooted in lived experience and personal agency—qualities not found in machine algorithms.
AI as a Tool, Not a Mind
Ultimately, even the most advanced LLMs can be viewed as tools, not true cognizers. They are designed to solve specified problems, lacking personal motivation or autonomous will. Humans, by contrast, integrate knowledge across domains—social, emotional, and intellectual—and challenge or refine what is learned.
The Essence of Human Autonomy
Whereas AI systems remain dependent on external programming and data, humans possess the freedom to choose their direction and redefine their goals. Whether guided by cultural beliefs, religious teachings, or personal experiences, human thought transcends data patterns by generating new ideas, empathizing with others, and embracing paradox or uncertainty.
Concluding Position: AI Cannot Truly Think
The debate over AI’s ability to “think” has persisted since Turing’s early inquiries. After examining both the richness of human cognition and the inherent constraints of AI, it appears that no large language model, artificial intelligence, or artificial general intelligence (AGI) can truly think. Genuine thought requires sentience, emotional depth, introspection, and the potential for contradictions that spark creativity. AI tools continue to evolve but remain fundamentally reliant on human input.
A Word of Caution: Preserving Human Agency
The genuine risk is not that AI might develop autonomous consciousness but that people may gradually cease to exercise their own cognitive faculties, deferring thinking entirely to machines. Human identity is rooted in a capacity for independent inquiry, creativity, and emotional engagement. Surrendering these qualities would diminish what it means to be human far more than any technological threat could.
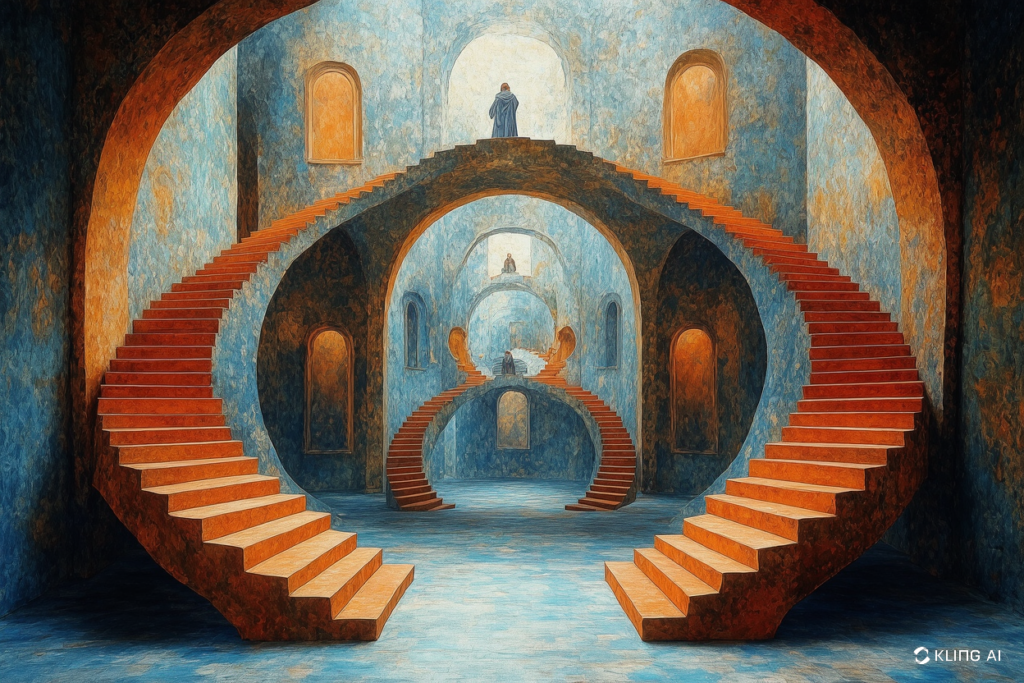